군용 차량 및 무인 로봇의 신뢰성 향상을 위한 다중 레이블 학습 기반 예측 정비(PHM) 시스템
A Multi-Label Learning-Based PHM System for Enhancing Reliability in Military Vehicles and Unmanned Robots
저자
박상혁, 박지혁
수록페이지
p.22-49 (28pages)
조회수
9
다운로드
0
- 창간연도
- 2024년 1월
- ISSN
- (Print)3022-6678 , (Online)3022-6643
- 수록권호
- 제2권 2호 (통권 6호)
- 발행일
- 2025.04
- 수록논문
- 2 articles
- 유형
- 학술저널
- 주제
- 사회과학, 자연과학, 예술체육학, 복합학
- 발행기간
- 2024.01 ~ 2025.04
- 발행주기
- 연 4회(계간)
- 총 권호 수
- 6 volumes
- 총 논문 수
- 37 articles
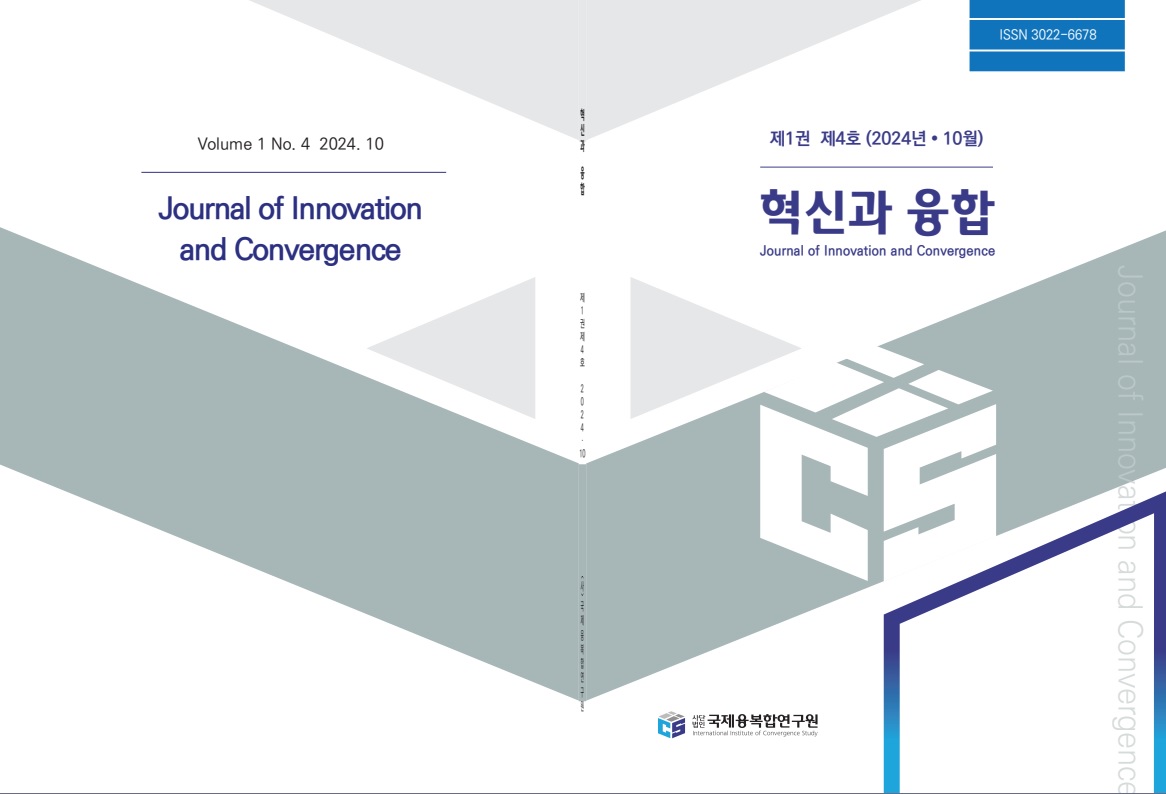
키워드
다중 레이블 학습, 예측 정비, 건전성 예측 및 관리, 군용 차량, 무인 로봇Multi-label learning, Predictive maintenance, Prognostics and health management, Military vehicles, Unmanned robots
초록
본 연구는 군용 차량과 무인 로봇의 신뢰성을 향상시키기 위한 다중 레이블 학습 기반 예측 정비 (PHM) 시스템을 제안한다. 혹독한 작전 환경에서 운용되는 군용 차량과 무인 로봇은 다양한 부품에서 복합적인 고장이 동시에 발생하는 경우가 많다. 기존의 단일 레이블 고장 진단 방식은 하나의 고장만 을 진단하는 구조로, 복합 고장 상황에서 일부 고장을 간과할 위험이 있다. 이에 반해 다중 레이블 학 습은 하나의 입력 데이터에 대해 여러 고장 레이블을 동시에 할당함으로써 중첩된 결함 상태를 포괄적 으로 진단할 수 있다. 본 연구에서는 센서 데이터 수집 및 전처리부터 다중 레이블 분류 기반 고장 진 단, 상태 예측, 정비 의사결정 지원까지 이어지는 통합 PHM 아키텍처를 제안한다. 또한 시뮬레이션 기반 실험 설계를 통해 제안된 시스템의 효과와 한계점을 분석한다. 다중 레이블 학습 기반 접근법은 복합 고장을 누락 없이 감지함으로써 고장 후 대응 비용과 예상치 못한 임무 중단을 줄이고, 군용 차량 및 무인 로봇의 운용 신뢰성을 향상시킬 것으로 기대된다.This study proposes a multi-label learning-based Prognostics and Health Management (PHM) system for enhancing reliability in military vehicles and unmanned robots. These systems, operated in harsh operational environments, frequently experience compound faults across various components simultaneously. Traditional single-label diagnostic models classify only one fault type at a time, thus risking overlooking some faults in compound failure situations. In contrast, multi-label learning assigns multiple fault labels to a single input, enabling comprehensive diagnosis of overlapping fault conditions. This research presents an integrated PHM architecture encompassing sensor data collection and preprocessing, multi-label classification-based fault diagnosis, state prediction, and maintenance decision support. The system’s effectiveness and limitations are analyzed through simulation-based experimental design. The multi-label learning approach is expected to detect compound faults without omission, thereby reducing post-failure response costs and unexpected mission downtime while improving operational reliability of military vehicles and unmanned robots.